Diferencias de desempeño académico con base en emociones en clases de matemáticas
DOI:
https://doi.org/10.22201/fesa.29928273e.2025.10.82Palabras clave:
Expresiones faciales, Geometría Analitica, desempeño matemático, teoría del control-valor, marco afectivo del aprendizaje.Resumen
El uso de tecnologías para analizar expresiones faciales con el fin de identificar emociones y mejorar el rendimiento en educación es común. El objetivo de este trabajo es comprender mejor el aprendizaje matemático en este contexto, en especial de la Geometría Analítica, considerando emociones y desempeño académico. Para ello, se emplean la teoría del control-valor y el marco afectivo para el aprendizaje como cuerpo teórico. Se realizó un estudio con 95 estudiantes, durante el cual se grabaron sus expresiones faciales y se extrajeron emociones, junto con mediciones objetivas y subjetivas de aprovechamiento. Los resultados muestran que, en mediciones objetivas, los estudiantes de alto desempeño experimentan más cambios emocionales que los de bajo desempeño; mientras que los últimos reportan una sensación de seguridad en sus habilidades y conocimiento en mediciones subjetivas. El texto concluye con algunas recomendaciones para mejorar el aprendizaje matemático.
Citas
Bossé, M., Bayaga, A., Lynch-Davis, K., & DeMarte, A. (2021). Assessing Analytic Geometry Understanding: Van Hiele, SOLO, and Beyond. International Journal for Mathematics Teaching and Learning, 1- 23.
Burroughs, E., Arnold, E., & Álvarez, J. (2023). Encountering ideas about teaching and learning mathematics in undergraduate mathematics courses. ZDM Mathematics Education, 55, 897- 907.
Doi:https://doi-org.pbidi.unam.mx:2443/10.1007/s11858-022-01454-3
Calero, C., Shalom, D., Spelke, E., & Sigman , M. (2019). Language, gesture, and judgment: Children’s paths. Journal of Experimental Child Psychology, 70-85. Doi:https://doi-org.pbidi.unam.mx:2443/10.1016/j.jecp.2018.07.015
Caratozzolo, P., Lara-Prieto, V., Hosseini, S., & Membrillo Hernández, J. (2022). The use of video essays and podcasts to enhance creativity and critical thinking in engineering. International Journal on Interactive Design and Manufacturing 16, 1231-1251.
Doi:https://doi-org.pbidi.unam.mx:2443/10.1007/s12008-022-00952-8
Csikszentmihalyi, M. (1996). Flow. Kairós.
Delahunty, T. (2023). International Encyclopedia of Education. Neurocognitive and physiological measurement of STEM learning processes, 458-471. Doi:https://doi-org.pbidi.unam.mx:2443/10.1016/B978-0-12-818630-5.13040-4
D’Mello, S., & Graesser, A. (2012). Dynamics of affective states during complex learning. Learning and Instruction, 22(2), 153-170.
Eckmann, J.P., Kamphorst, O., Oliffson, S., & Ruelle, D. (1987). Recurrence Plots of Dynamical Systems. Europhysics Letters, 4(9), 973.
Eligio, U. (2017). An Overview of the Growth and Trends of Current Research on Emotions and Mathematics. In U. Eligio, Understanding Emotions in Mathematical Thinking and Learning (pp. 3 - 41). Academic Press.
Doi:https://doi-org.pbidi.unam.mx:2443/10.1016/B978-0-12-802218-4.09994-5
Geetha, A., Mala, P., & Uma, E. (2023). Multimodal Emotion Recognition with Deep Learning: Advancements,challenges, and future directions. Information Fusion, 2-38. Doi:https://doi.org/10.1016/j.inffus.2023.102218
Harackiewicz, J. (2002). Predicting sucess in college: a longitudinal study of achievement goals and ability measures as predictors of interest and performance from freshman year through graduation. Journal of Education Psychology, 94(3), 562 - 575.
Jamaludin, N. L., Mohd Nasir, J. S., Isa, S. S. y Ali, A. (2023). @INNOVATIVEESCAPE: Students’ perception and perceived learning outcome using an online exhibition via Instagram platforms. Environment - Behaviour Proceedings Journal, 9(SI17), 471- 478. https://doi.org/10.21834/ebpj.v9iSI17.5453
Karabenick, S. (1991). Relationship of academic help seeking to the use of learning strategies and other instrumental achievement behavior in college students. Journal of Educational Psychology, 83(2), 221-230.
Miragliotta, E. (2022). Geometric prediction: A framework to gain insight into solvers’ geometrical reasoning. The Journal of Mathematical Behavior, 65, 15. Doi:https://doi-org.pbidi.unam.mx:2443/10.1016/j.jmathb.2021.100927
Montgomery, D. (2017). Design and Analysis of Experiments. John Wiley.
Ng, O., Shi, L., & Ting, F. (2020). Exploring differences in primary students’ geometry learning outcomes in two technology - enhanced environments: dynamic geometry and 3D printing. International Journal of STEM Education, 50(7).
Doi:https://doi.org/10.1186/s40594-020-00244-1
Pekrun, R., Elliot, A., & Maier, M. (2009). Achievement goals and achievement emotions: A meta - analysis. Educational Psychology Review, 21(4), 359 -386.
Pise, A. A., Vadapalli, H., & Sanders, I. (2022). Estimation of Learning Affects Experienced by Learners: An Approach Using Relational Reasoning and Adaptive Mapping. Wireless Communications and Mobile Computting, 14. Doi:https://doi.org/10.1155/2022/8808283
Prakash, J., Van Haneghan, W., Blackwell, S., Murugesan, D., Tamilselvan, G., & Tamilselvan. (2019). Classroom engagement evaluation using computer vision techniques. Pattern Recognition and Training XXX, 192 -199.
Sharma, K., Papavlasopoulou, S., & Giannakos, M. (2022). Children’s facial expressions during collaborative coding: Objective versus subjective performances. International Journal of Child - computer Interaction, 34, 1- 20. Doi:https://doi.org/10.1016/j.ijcci.2022.100536
Spector, J., Lockee, B., & Childress, M. (2023). Learning, Design, and Technology. An International Compendium of Theory, Research, Practice, and Policy. Springer Nature.
Tze, V., Parker, P., & Sukovieff, A. (2022). Control - Value Theory of Achievement Emotions and its Relevance to School Psychology. Canadian Journal of School Psychology, 37(1), 23 - 39. Doi:https://doi.org/10.1177/08295735211053962
Weisberg, S. M. & Newcombe, N. S. (2017). Embodied cognition and STEM learning: overview of a topical collection in CR:PI. Cognitive Research: Principles and Implications, 38(2), 2- 6. Doi: 10.1186/s41235-017-0071-6
Woolf, B., Betke, M., Yu, H., Bargal, S., Arroyo, I., Magee, J., Allesio, D., & Rebelsky, W. (2023). FACE READERS: The Frontier of Computer Vision and Math Learning. AIED 2023 Workshop: Towards the Future of AI -Augmented Human Tutoring in Math Learning (pp. 1-13). CEUR Workshop.
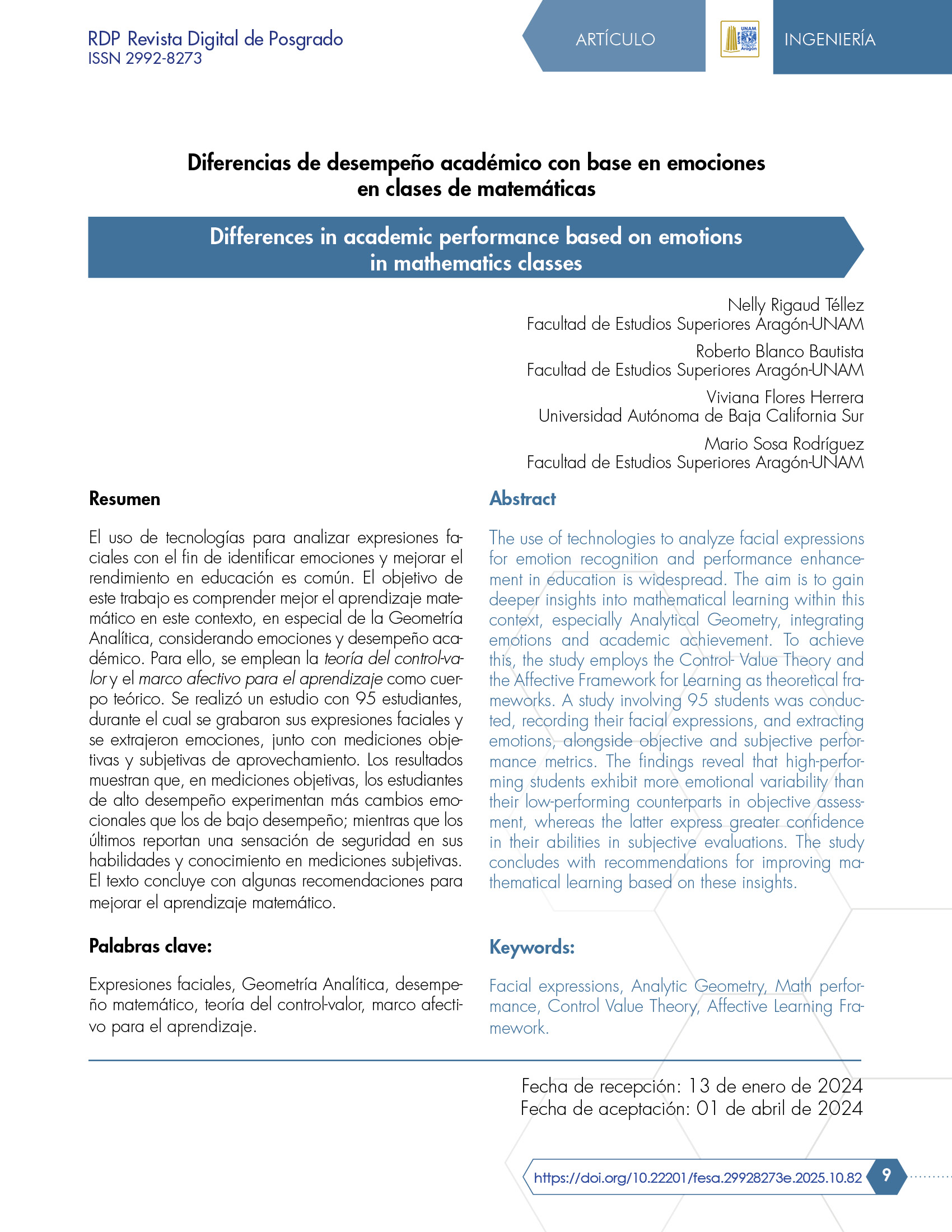
Descargas
Publicado
Cómo citar
Número
Sección
Licencia
Derechos de autor 2024 Universidad Nacional Autónoma de México

Esta obra está bajo una licencia internacional Creative Commons Atribución-NoComercial-SinDerivadas 4.0.